The internet is rife with myths and articles making dubious claims about certain foods and their anti-cancer properties. We have all seen the articles of questionable scientific merit gracing social media suggesting that such-and-such foods can cure cancer, the majority of which are highly questionable. A new study offers a unique kind of insight into the potential true effectiveness of food in fighting cancer [1].
Investigating molecules in food with machine learning
There is no doubt that there are many foods that contain a myriad of active molecules, and perhaps some of these food myths may have a grain of truth to them. A team of researchers decided to do some real myth-busting and put a variety of bioactive molecules found in foods to the test to see if they might potentially help to combat cancer.
The research team chose to use the power of machine learning to help assess a total of 7,962 biologically active compounds encountered in dietary sources. These molecules were compiled into a database and fed into a machine learning algorithm, which determined that of these compounds, there were 110 molecules that appeared to have anti-cancer properties.
Machine learning is ideally suited to the kinds of laborious and time-intensive tasks in which a large volume of data must be accurately examined in a standard way. Machine learning can also sometimes spot correlations missed by researchers, so it is rapidly becoming a highly valuable tool in the researcher’s toolkit.
With their findings, the researchers then produced a ‘food map’ that showed the value of different foods based on their cancer-beating potential. On the map, each circular node represents a particular food; the larger the circle, the more anti-cancer molecules the particular food contains. You can also see interconnecting lines between nodes; this shows when connected foods contain a similar range of molecules and allows foods to be grouped by molecular commonality.
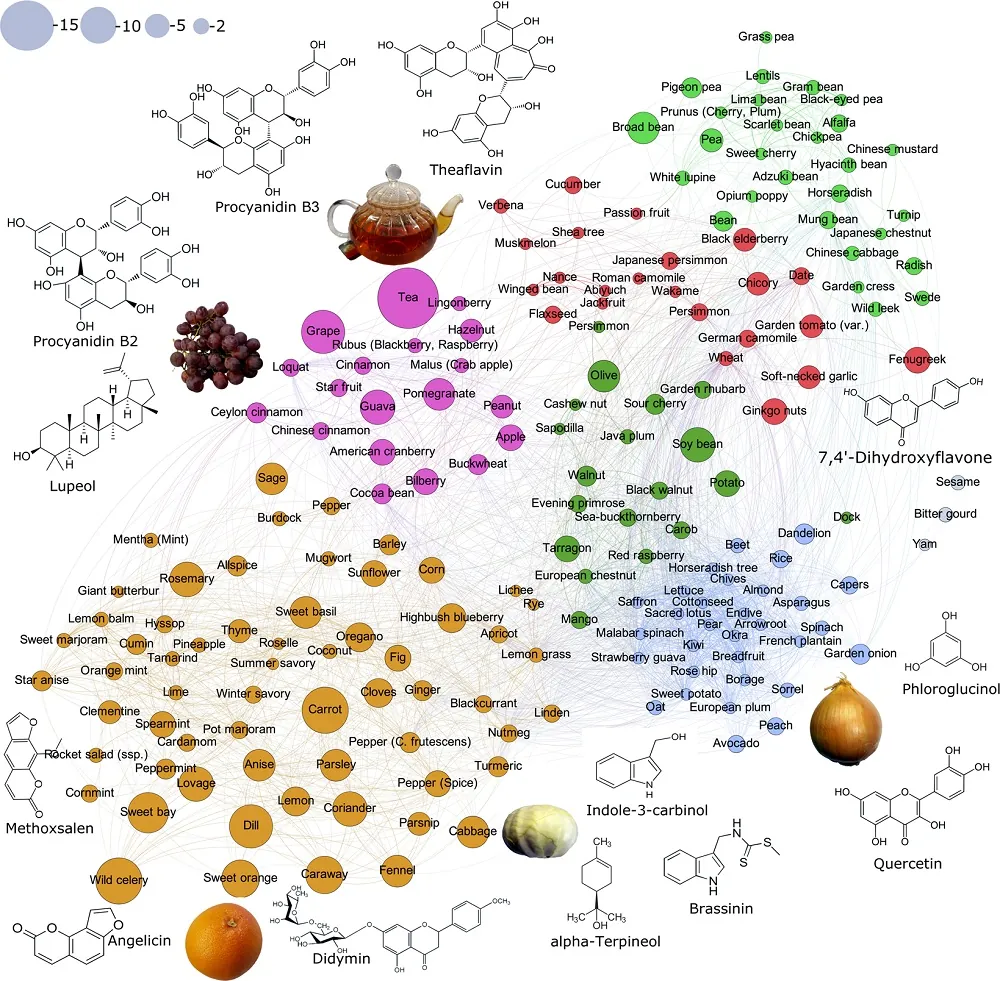
Recent data indicate that up-to 30–40% of cancers can be prevented by dietary and lifestyle measures alone. Herein, we introduce a unique network-based machine learning platform to identify putative food-based cancer-beating molecules. These have been identified through their molecular biological network commonality with clinically approved anti-cancer therapies. A machine-learning algorithm of random walks on graphs (operating within the supercomputing DreamLab platform) was used to simulate drug actions on human interactome networks to obtain genome-wide activity profiles of 1962 approved drugs (199 of which were classified as “anti-cancer” with their primary indications). A supervised approach was employed to predict cancer-beating molecules using these ‘learned’ interactome activity profiles. The validated model performance predicted anti-cancer therapeutics with classification accuracy of 84–90%. A comprehensive database of 7962 bioactive molecules within foods was fed into the model, which predicted 110 cancer-beating molecules (defined by anti-cancer drug likeness threshold of >70%) with expected capacity comparable to clinically approved anti-cancer drugs from a variety of chemical classes including flavonoids, terpenoids, and polyphenols. This in turn was used to construct a ‘food map’ with anti-cancer potential of each ingredient defined by the number of cancer-beating molecules found therein. Our analysis underpins the design of next-generation cancer preventative and therapeutic nutrition strategies.
Conclusion
Many foods appear to contain molecules that can potentially have an effect on cancer. This study goes a good way towards dispelling food myths by applying the scientific method to investigate these molecules and is another example of how machine learning is helping research.
Of course, foods are not a substitute for professional healthcare in the effective treatment of cancer, but they could potentially be a useful co-therapy provided that their efficacy can be determined.
Literature
[1] Veselkov, K., Gonzalez, G., Aljifri, S., Galea, D., Mirnezami, R., Youssef, J., … & Laponogov, I. (2019). HyperFoods: Machine intelligent mapping of cancer-beating molecules in foods. Scientific reports, 9(1), 9237.